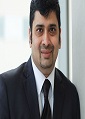
Sri Krishnan
Ryerson University, Canada
Title: Digital technologies and machine learning for ubiquitous monitoring of neurological and neuromuscular disorders
Biography
Biography: Sri Krishnan
Abstract
Many neurological and neuromuscular disease management and screening could benefit from long-term processing and analysis of physiological signals such as EEG, EMG, EOG, PSG, speech, and gait rhythm. The advancements in wearable digital health technologies and the availability of massive storage and computational processing power has made it possible for implementation of advanced mathematical and signal processing techniques for pre-processing tasks such as removal of noise and reduction of interferences/artifacts, extraction of robust features/signatures from physiological signals, and implementing machine learning systems for informed clinical decision making. For example, signal analysis research (SAR) group at Ryerson University has analyzed gait rhythm for automatic classification of Parkinson’s, Huntington’s and ALS. We have also processed and analyzed the non-stationary nature of polysomnographic sleep signals in identifying RBD disorders and PLMS. The group is also involved in processing and analyzing sensor information related to EOG and voices in identifying signal features related to diseases such as Parkinson’s and sleep related disorders. The challenges associated in these areas are that the signals acquired are non-stationary, produced by non-linear systems, and have variabilities associated with them they could be analyzed to provide rich information in temporal, spectral or spatial domains by using advancements of signal processing and machine learning. The cost effective and patient comfort associated with human factors make them valuable for home-based, telehealth and mobile health based long-term data acquisition and monitoring application. The exponential growth in digital technologies related to sensors and communications (For example, Internet of Things-IoT), and the availability of data and machine learning/AI techniques are all poised to make the field of clinical decision making and patient management even more affordable and ubiquitous. In any of these digital healthcare designs, security and privacy protocols have to be incorporated as part of the design process pipeline.